A software system is being developed at the AIT Austrian Institute of Technology that bundles indications of fake texts, images, audio signals and videos and uses them to produce an overall assessment of the truthfulness of a message.
The spread of disinformation is a serious threat: fake news influences decisions, leads to uncertainty, the build-up of public discontent and the destabilization of society and democracy. In times of crisis, such as the current Covid 19 pandemic, disinformation has reached a new negative peak; both governmental organizations and media companies, as well as each individual citizen, are facing major challenges for which there are currently hardly any suitable answers or effective countermeasures.
Meanwhile, research is making progress to combat the uncontrolled spread of disinformation while respecting social, cultural and legal norms. New methods based on artificial intelligence (AI) and the aggregation of information from different sources should make it possible to automatically verify the truth of news in the future. However, the road ahead is still rocky, and high hurdles still need to be overcome in many areas.
Videos from computer
One hotly debated area at present is "deep fakes", i.e. manipulated videos in which the people involved have been replaced or statements are put into their mouths that they never said. The basis for faked images are so-called "Generative Adversorial Networks" (GAN). These are two deep-learning systems coupled together: a "generator" artificially creates images, and a "discriminator" tries to classify them as genuine or false. In many rounds, the generator learns to fake better images, and the discriminator learns to classify them correctly. "The two artificial intelligences train each other," explains Ross King, head of the Data Science & Artificial Intelligence Competence Unit at AIT.
Something similar can be applied to videos. In the "face swapping" process, an AI system learns to reconstruct the face of person A. Another system analyzes movement patterns of person B. In the next step, A's face is linked to B's movement style ("style transfer"), creating deceptively real videos of situations that never existed in reality. "The software for this is available for free on the web, and there are even good instructions that you just have to follow step by step," reports Alexander Schindler, a fake news expert and data scientist at AIT.
However - and this is the good news - this method for high-quality fakes is very complex and therefore not yet suitable for mass production. The famous fake videos of top politicians are the result of professional productions by highly specialized teams, explains the researcher.
Virtual faces do not blink
One of the difficulties with this is that there is not yet a way to put any text into the mouth of a particular voice (see below for details). In the well-known deep fake videos, voice imitators have been hired to speak a precisely planned text that matches the gestures and posture of the person whose face is being replaced.
Another problem arises when there are not enough images from all walks of life for the computer-generated faces to train the AI on. This is especially true of "avatar methods," in which the original face is manipulated by facial movements of a second actor. For example, for most people of public interest, there are hardly any pictures in which they have their eyes closed. As a result, the faces in deep fake videos don't blink - and that's very easy to detect. Moreover, if there are only a few training images for the AI, the skin structure is displayed too smoothly and rubbery, unnatural distortions occur. This can also be detected relatively easily.
AI methods now add to the traditional methods of image forensics (such as analyzing metadata, shadows in relation to light sources, or image noise). "The new methods are more adaptive and are much better at explaining where they detected manipulations and the nature of the manipulations," Schindler said. Moreover, he said, conclusions can be drawn about the source images. "In generated content, AI is now fighting AI," the expert summarizes.
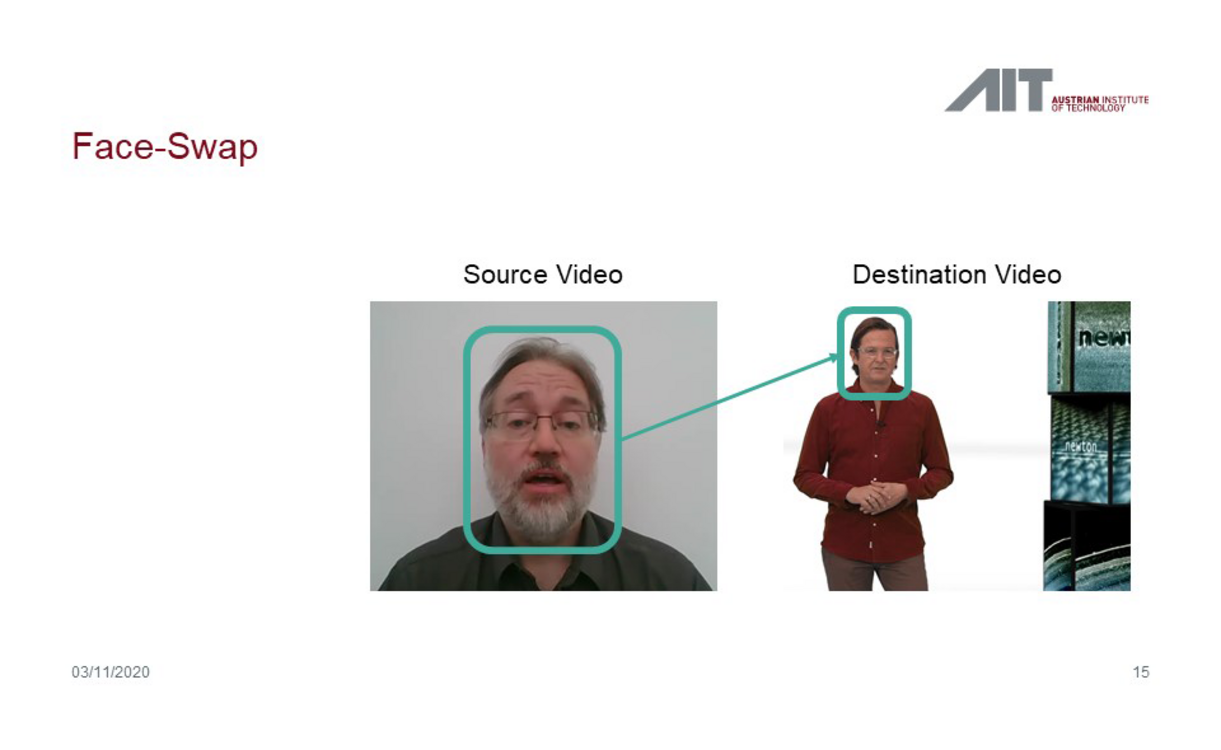
This is how a deep fake is created using the "face swapping" method: In the first step, the computer is trained to reconstruct the face of a person. The same is done with the body of a second person. Finally, in the last step, the face of the first person is copied into the video of the second person. Here, as an example - created for the ORF documentary "Newton" - the face of the presenter was exchanged for that of AIT researcher Ross King.
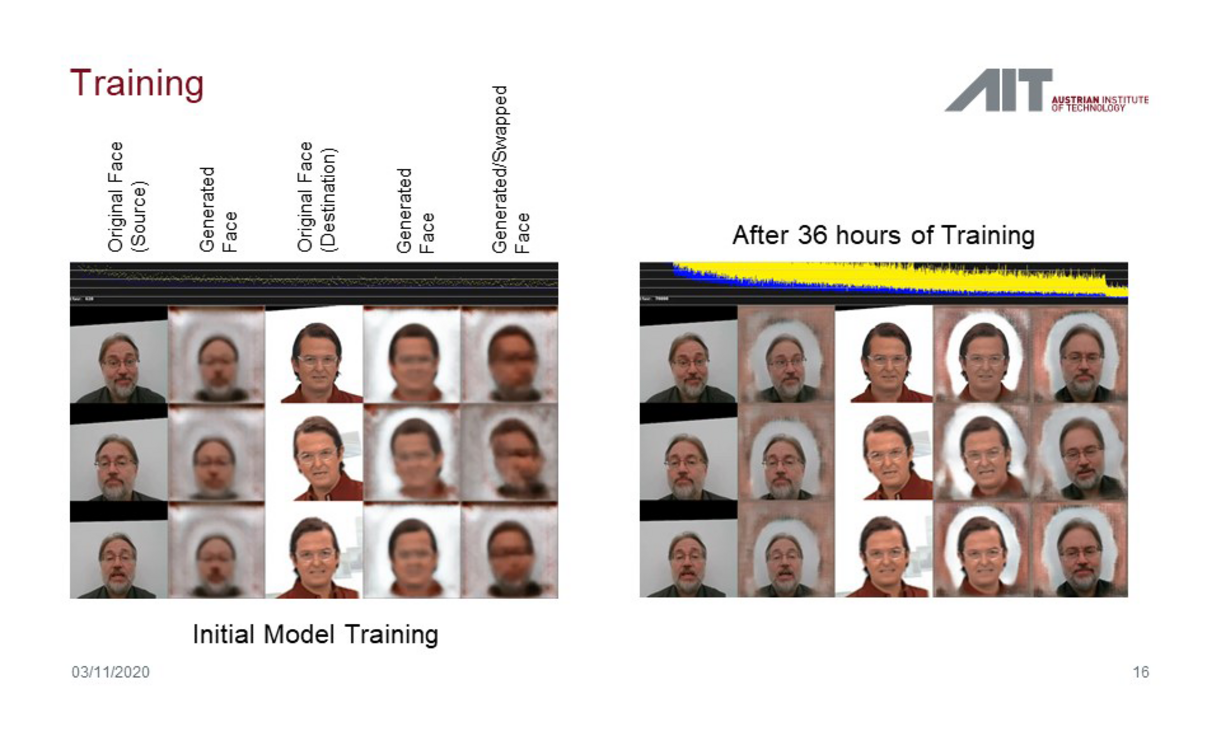
This is how a deep fake is created using the "face swapping" method: In the first step, the computer is trained to reconstruct the face of a person. The same is done with the body of a second person. Finally, in the last step, the face of the first person is copied into the video of the second person. Here, as an example - created for the ORF documentary "Newton" - the face of the presenter was exchanged for that of AIT researcher Ross King.
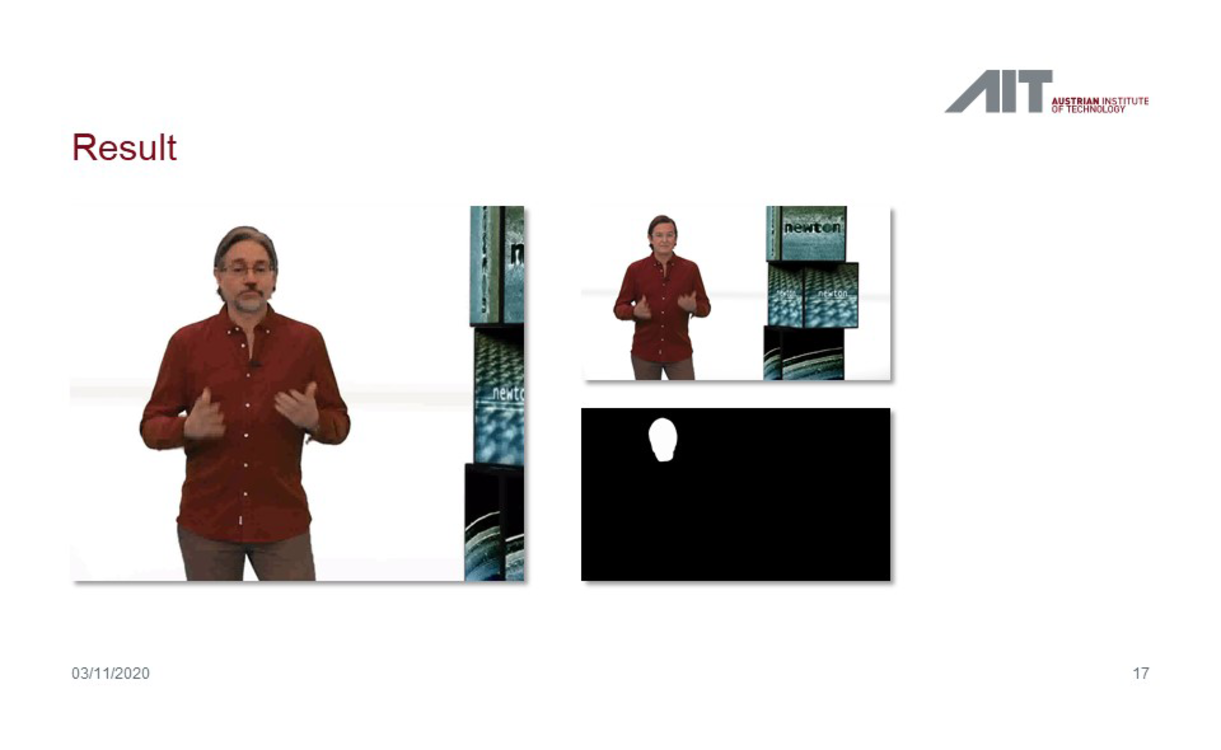
This is how a deep fake is created using the "face swapping" method: In the first step, the computer is trained to reconstruct the face of a person. The same is done with the body of a second person. Finally, in the last step, the face of the first person is copied into the video of the second person. Here, as an example - created for the ORF documentary "Newton" - the face of the presenter was exchanged for that of AIT researcher Ross King.
Voices can hardly be faked
As mentioned, audio data is not yet as advanced as images. "Currently, it is still very difficult to artificially generate truly natural voices," says Schindler. The main reason for this is that we are much more sensitive to hearing than to seeing. "We are now used to images being noisy or having low resolution and being pixelated. With speech, we're much more skeptical about that." Add to that the fact that noise manifests itself differently with audio signals: The voice sounds metallic, you hear phase shifts and the like.
As a result, there are still no satisfactory algorithms for, say, a style transfer of audio files (whereby speech rhythm, speech melody or voice pitch could be adapted to a target person). "In this area, we still have a head start on producers of fake news," says Schindler. However, he expects some progress in this field as well over the next few years. Training data for AI systems for this exists in any case: audio books in which for every written word there is also a spoken variant.
Counterfeit audio signals can - at present - be detected well with conventional methods of audio forensics. This ranges from analyzing the network frequency of the current on which a recording device is connected, to classifying the microphone, to analyzing the phasing of sound vibrations (which can be used to detect cuts, for example). In the future, AI systems will help detect fakes in audio as well.
Automated fact-checking still in its infancy
Finding fake news in texts is much more difficult. "So much content is conveyed here that requires general knowledge that detecting false information is very difficult for the computer," says Schindler. Manual fact-checking therefore still works best. But this can only be done on an ad hoc basis - "you can't use it to check millions of Internet pages for false reports."
Automatic fact-checking is still in its infancy. "For the computer, a text is not knowledge, but just words strung together," the researcher says, outlining the basic problem. "In language, single words often make big differences. With hate speech, satire or sarcasm, you go into extremely complex subtle subcontexts." Matching facts with databases such as Wikpedia, for example, therefore works at most with very formal language, he said. With the help of AI, words can be put into context more and more effectively in the process. "The big challenge is how to reference different data sets," Schindler said.
How does fake news work?
That is why the researchers are also pursuing other approaches. The analysis of how users react to messages in social networks ("stance detection") is promising. Is a message received positively? Does a controversy erupt? Are there pronounced echo chambers? And more fundamentally, how does fake news spread on social networks? "If you put all this together - the spread, people's reactions and the content analysis - you already get very good results," Schindler reports. In experiments at AIT, this allowed around 85 percent of fake news in articles to be correctly classified.
However, it is not enough to classify a news item as a whole as correct or incorrect - more detailed information is needed about which parts of a text are incorrect, explains Ross King. To this end, the experts at AIT apply methods of so-called "explainable AI". This involves analyzing how an AI arrives at the decision "true" or "fake" - in other words, what factors have led to such a classifier. For example, a series of experiments at AIT examined which keywords led to the classification of a text as discriminatory. "It was nice to see that the words Machine Learning responds to overlap with keywords from a linguistic analysis," Alexander Schindler reports.
A "nutrition table" for texts
Based on such findings, a system is to be constructed that flags critical words in texts and also suggests Internet links where suspicious passages can be cross-checked. Schindler has in mind something like a "nutritional value table" for an article, which says something about the truth content and the degree of polarization and provides information about whether an article is fact-based or rather emotionalizing.
"We need helpful tools like this," Ross King sums up. These would also contribute to a higher awareness among users to be more skeptical about content on the Internet and to take a closer look at its origin ("digital litteracy").
Overall interpretation
Researchers are now going one step further: In order to recognize "fake news" even more accurately, they want to combine the analysis results from the different areas - images, video, audio, texts - to enable a holistic interpretation. "The difficult thing is that it's not so easy to combine the different modalities," explains Alexander Schindler. This is where an AI-based fusion logic is supposed to help.
Such a system, which combines classical and AI-driven approaches, is currently being developed in a project under the Austrian security research program KIRAS. At the end of this two-year research project, there should be an AI-based fake news detection system that is tailored to the respective end users and can be used in the daily work environment. On the one hand, this should enable users to make an initial assessment of the credibility of content and, on the other hand, provide public authorities with opportunities to take swift action.
Related link
IDSF VIENNA 2020
The topic of fake news will also play a central role at the International Digital Security Forum (IDSF), which will be held as a hybrid conference in Vienna on December 2 and 3. The motto of this event, organized by the AIT Austrian Institute of Technology and the Austrian Federal Economic Chamber (WKO), is "Security in times of pandemics and major global events". Sessions are planned on topics including border management, cyber crime, situation awareness, fake news and digital resilience. The aim of the IDSF is to strengthen the cooperation between research, private and public sector for the development and application of technologies for socially desirable developments.